In the world of SaaS, “efficiency” isn’t just a buzzword—it’s the difference between scaling fast and stalling out. Every founder and product manager eventually faces a big question: Should we lean into AI, or focus on automation?
They sound similar. They often overlap. But they are not the same. And depending on your goals, stage, and team, the right choice can look very different.
So let’s break it down.
Related Article: https://www.adlabz.co/the-role-of-ai-in-b2b-saas-how-automation-is-changing
Jump to:
What’s the Difference Between AI and Automation?
Let’s start with the basics.
Automation is about rules.
It’s deterministic. You define a set of steps (“if this, then that”), and machines follow them—faster, more reliably, and at scale.
Examples:
- An email goes out when a trial ends.
- A CRM record updates when someone clicks a link.
- Your support ticket system assigns cases based on priority.
AI is about learning.
It’s probabilistic. Instead of following hard-coded rules, it adapts to data patterns and makes decisions, often improving over time.
Examples:
- A chatbot that understands natural language.
- A model that predicts churn before it happens.
- Dynamic pricing that adjusts based on user behavior.
Both aim to boost productivity, but they serve very different purposes.
When Does Automation Make More Sense for SaaS?
Are your tasks repetitive and rule-based?
If you’re dealing with a high volume of predictable, repeatable tasks, automation is your best friend.
Use cases where automation shines:
- Lead routing: Assigning leads based on geography or plan type.
- Billing workflows: Sending payment reminders, invoices, or access locks.
- Marketing ops: Triggering welcome emails or onboarding sequences.
These are scenarios where outcomes are known and variables are minimal. You don’t need learning—you just need reliable execution.
Do you want transparency and control?
Automation is more “explainable.” You can audit exactly what happened and why, and change the logic.
That’s a big win in industries like fintech, healthcare, or legal tech, where compliance matters.
Example:
A SaaS like Chargebee uses automation to manage recurring billing, dunning emails, and tax compliance—all without relying on AI. It’s faster, cheaper, and easier to maintain.
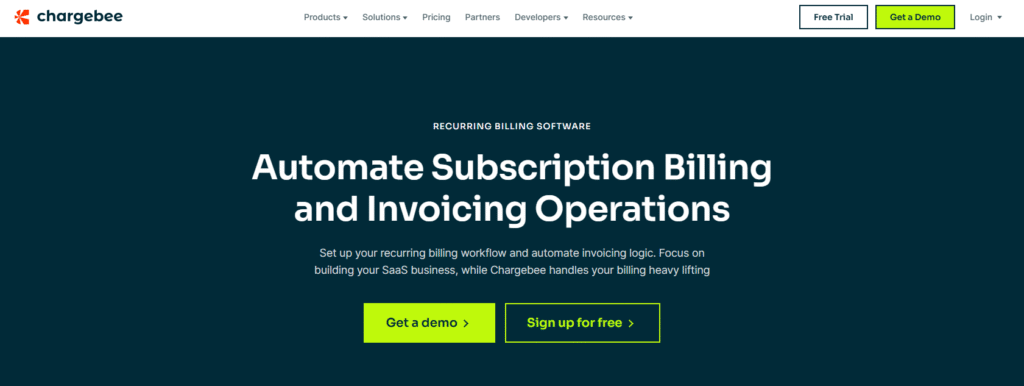
When Should SaaS Companies Choose AI Instead?
Is your data messy, unpredictable, or human-like?
AI is powerful when data doesn’t follow rules. Human speech. Behavior. Images. Preferences. These can’t be captured with simple logic trees.
Great AI use cases in SaaS:
- NLP (Natural Language Processing) for customer support or knowledge bases.
- Forecasting user churn, LTV, or trial-to-paid conversions.
- Personalizing experiences based on user behavior.
Are you trying to learn from users, not just respond to them?
AI is great at finding patterns humans miss. This makes it ideal for personalization, optimization, and prediction.
Example:
Intercom uses AI-powered bots that go beyond answering FAQs—they can interpret customer intent and escalate intelligently, improving resolution time and user satisfaction.
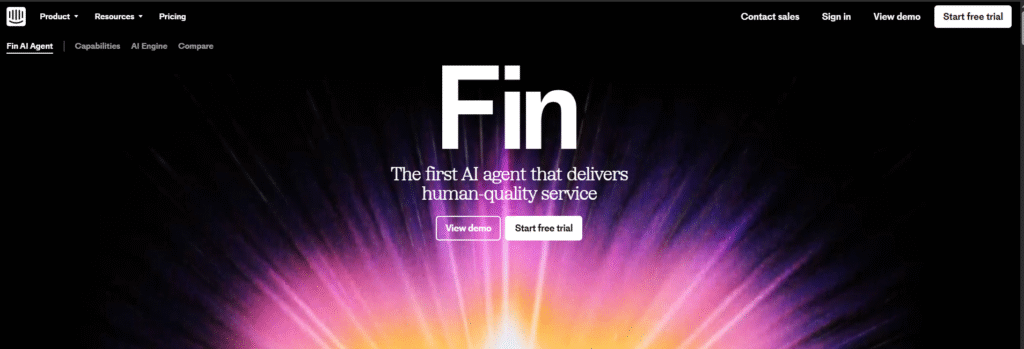
How AI Can Improve Customer Support in SaaS
Customer support is one of the most common—and most powerful—areas to apply AI in SaaS.
AI applications in support:
- Chatbots that can handle level-1 queries, freeing up your human team.
- Sentiment analysis to prioritize angry or urgent messages.
- Auto-tagging and routing tickets based on language and issue type.
Result?
Faster responses, better scalability, and fewer support tickets slipping through the cracks.
Case in point:
Zendesk introduced AI-powered bots in their platform, allowing customers to build intelligent workflows that deflect tickets before they even reach a human.
What About Cost, Complexity, and Time to Value?
Let’s get practical.
Factor | Automation | AI |
---|---|---|
Implementation | Simple, often no-code | Complex, often requires engineering/data |
Time to Value | Fast (days to weeks) | Slower (weeks to months) |
Maintenance | Low (until logic changes) | Medium to High (requires retraining/tuning) |
Cost | Lower (tools like Zapier, Make, HubSpot) | Higher (custom models, APIs, infrastructure) |
Scalability | High, but limited by complexity | Extremely scalable once trained |
For early-stage SaaS or lean teams, automation often delivers faster ROI. But as you grow and collect more data, AI can unlock strategic advantages.
Can You Use Both? (Spoiler: Yes)
The smartest SaaS teams don’t pick one over the other—they combine both.
Think of automation as the skeleton and AI as the brain.
You can automate data flows and user journeys and inject intelligence into key touchpoints. For example:
- Automate a customer onboarding sequence.
- Use AI to personalize the sequence based on user persona or industry.
- Feed insights back into the loop using predictive analytics.
This hybrid approach keeps operations smooth and user experiences smart.
Top Mistakes SaaS Companies Make When Choosing AI or Automation
- Using AI when a rule would work better
Sometimes, a simple “if/then” logic is more efficient than a model. Don’t over-engineer. - Underestimating the data required for AI
AI needs training data. If you don’t have enough, you’ll end up with underperforming models. - Ignoring maintenance needs
Automation may require occasional logic updates. AI may need model retraining. Both need ongoing oversight. - Failing to measure impact
Many SaaS companies implement tools but never benchmark before vs after. Always tie efforts to KPIs.
What Tools Should SaaS Teams Use
Automation Tools
- Zapier / Make / n8n – Connect apps and automate workflows without code.
- HubSpot Workflows – Automate marketing, sales, and support actions.
- Tray.io / Workato – For more complex enterprise automation.
AI Tools
- OpenAI / Anthropic – Build custom GPT agents for support or content.
- Pinecone / Weaviate – Vector databases for building recommendation engines.
- Google Vertex AI / AWS SageMaker – For deploying and managing custom models.
- ChurnZero / Pendo / Amplitude – AI-powered product analytics and predictions.
Checklist: How to Evaluate Whether AI or Automation Fits Your Use Case
- Is the task rule-based and repeatable? → Automation
- Do you need human-like interpretation (text, voice)? → AI
- Do you have clean, labeled data to work with? → AI
- Is explainability critical (e.g., legal workflows)? → Automation
- Are you working with time-sensitive, high-volume tasks? → Both, but start with Automation
- Do you need real-time learning and recommendations? → AI
Real-World Example: Notion
Notion started as a highly manual productivity tool. But over time, they layered in both automation and AI.
- Users can now automate workflows via templates and integrations.
- Their “Notion AI” features allow summarizing notes, writing docs, and extracting insights—all within your workspace.
This combo allows users to move faster and think smarter, without ever leaving the app.
That’s the power of integrating both.

The Future: Will AI Replace Automation
Short answer: No.
Long answer: AI will continue to make automation smarter, but rules-based automation is here to stay.
Here’s what we expect:
- More AI-powered automation tools that offer “intelligent suggestions” for workflow design.
- No-code AI platforms that let marketers or ops teams train small models without engineers.
- Blended experiences where automation does the execution and AI handles the decision-making.
Think of it like this: automation builds the engine, AI fine-tunes how it drives.
Summary Table: AI vs. Automation for SaaS
Dimension | Automation | AI |
---|---|---|
Primary Use Case | Repetition, speed | Prediction, adaptation |
Best For | Ops, CRM, support workflows | Personalization, insights, recommendations |
Data Need | Minimal | High |
Human-Like Input? | No | Yes (language, images, behaviors) |
Effort to Deploy | Low | Medium to High |
Risk/Black Box | Low (rule-based) | Higher (can be opaque) |
Examples | Stripe + Zapier = Auto-billing | Intercom AI bot, Notion AI, Jasper |
Case Study: How Jasper Uses AI to Personalize at Scale
Jasper, an AI-powered copywriting SaaS, has scaled rapidly by putting AI at the core of its value proposition. But beneath the surface, their success is a blend of intelligent automation and adaptive AI models.
Here’s how it works:
- Users input a basic prompt (e.g., “Write a blog about SaaS marketing”).
- Jasper’s AI engine (built on top of OpenAI) generates high-quality copy, but it doesn’t stop there.
- The system learns from user behavior—how often people edit certain phrases, which tone settings they prefer, and which templates convert best.
This data feeds into AI models that personalize outputs for specific industries, personas, and formats. On top of that, Jasper uses automation to trigger template suggestions based on content type and user history.
Key takeaway:
Jasper succeeds by using AI where creativity and nuance are needed and automation to speed up user flows. It’s a model many SaaS companies can replicate: creative intelligence powered by invisible systems.
AI and Data Privacy in SaaS: What Founders Need to Know
With AI comes responsibility, especially when dealing with customer data. SaaS platforms often handle sensitive information, and AI introduces additional layers of complexity.
Here are the key issues:
1. Data Collection Transparency
AI needs data to train and improve. But collecting user data—especially behavioral or textual—requires informed consent. Ensure your privacy policy clearly states:
- What data is collected
- How it’s used
- Whether it’s shared with third parties (e.g., model providers like OpenAI)
2. Data Storage & Retention
SaaS founders must know where data is stored. Are you using regional data centers? How long do you keep training data? Do users have the option to delete it?
3. Bias and Fairness
If your AI makes decisions (like scoring leads or predicting churn), you need to verify it’s not introducing bias. Regularly audit your models to ensure fairness across demographics or industries.
4. Compliance Requirements
If you’re selling in Europe, GDPR applies. In California, it’s CCPA. AI does not get a free pass—your usage must still comply with regional data laws.
Best practices:
- Use anonymization wherever possible.
- Offer opt-outs for AI-based personalization.
- Include an AI disclosure in onboarding or usage.
Ignoring these can lead to fines, customer mistrust, or brand damage. But handled correctly, it can be a trust builder that sets you apart.
5 Common Myths About AI and Automation in SaaS
Let’s bust some myths that hold SaaS founders back.
1. “AI is only for big companies.”
Wrong. Thanks to API-based models (OpenAI, Google, Anthropic), any SaaS can now integrate AI. Tools like Bubble and Zapier also offer AI blocks for no-code builders.
2. “Automation is outdated.”
Not even close. Automation isn’t going anywhere—it’s just being enhanced by AI. Think of AI as the decision-maker and automation as the executor.
3. “We don’t have enough data to use AI.”
You don’t need a huge dataset to get started. Pre-trained models are incredibly capable. You can fine-tune later once you collect proprietary data.
4. “AI will make our app confusing for users.”
Only if you overdo it. Done well, AI should make the experience feel smoother, not more complex. Tip: Start with simple enhancements like autofill or smart suggestions.
5. “You have to choose between AI and automation.”
False. The best SaaS platforms combine both. Start with automation, and add AI where it enhances decision-making or experience.
Bonus: Questions to Ask Vendors When Evaluating AI or Automation Tools
Not all tools are created equal. Before choosing a platform, ask these:
For Automation Vendors:
- Can non-technical users manage the workflows?
- Does it integrate with our current stack (CRM, analytics, support tools)?
- What happens if a step fails—are there fallback paths or alerts?
For AI Vendors:
- What’s the underlying model? Is it fine-tuned or open-source?
- How do you handle data privacy and storage?
- Can we audit decisions or outputs for transparency?
- What’s the cost model—per request, per user, or model?
These questions help filter hype from actual value and prevent vendor lock-in.
Final Thoughts: What’s Right for You?
If you’re still early-stage, trying to move fast without breaking the bank, start with automation. It’ll save time, reduce errors, and get things running smoothly.
But if you’re sitting on piles of behavioral data, facing scale issues, or needing personalization at scale, AI is worth exploring. Even small AI additions (like GPT-powered chat or smart tagging) can create a major impact.
And remember: you don’t have to go all-in on day one.
You can start with automation, then let AI enhance your workflows as you grow.
The real win comes when your SaaS is efficient and intelligent.
You might also be interested in: